

Inspect the folder associated with your project if you wish. R, I used toyline.R and note that, by default, it will go in the directory associated with your project. Now you have a new pane containing a nascent R script. Select these commands, skipping any that didn't work or contained typos. Visit the History tab of the upper right pane. Let's say this is a good start of an analysis and you're ready to preserve the logic and code. Y <- a + b * x + rnorm( 40, sd = sqrt(sigSq))
#Difference between r and rstudio pdf#
To emulate a real analysis, let's write a numerical result to file for later use - the average of the \(x\)'s - and let's save a scatterplot to PDF - a scatterplot of \(y\) versus \(x\) with the true data-generating line superimposed.
#Difference between r and rstudio plus#
I'm going to set the intercept \(a\) and slope \(b\) of a line, generate some \(x\) values uniformly on the interval \(\), and finally generate \(y\) values as \(a + bx\) plus some noise from a Gaussian distribution. Let's enter a few commands in the Console, as if we are just beginning an analytical project. I won't print my output here because this document itself does not reside in the RStudio Project we just created and it will be confusing. Now verify that the directory associated with your project is also the working directory of our current R process: getwd() Call it whatever you want (but bear in mind that good names are short and informative). The directory name you choose here will be the project name. Do this: Projects menu -> Create project. Let's make one to use for the rest of this tutorial. Keeping all the files associated with a project organized together - input data, R scripts, analytical results, figures - is such a wise and common practice that RStudio has built-in support for this via it's projects. A way that also puts you on the path to managing your R work like an expert. Or within the Files pane, choose More and Set As Working Directory.īut there's a better way. x Set Working Directory -> To Files Pane Location. Make an assignment and then inspect the object you just created. Go into the Console, where we interact with the live R process.


Suppose we use the merge() function in base R to perform a left join, using the ‘team’ column as the column to join on: #perform left join using base R
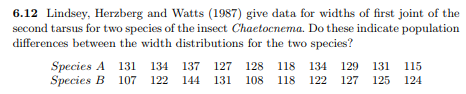
frame(team=c(' Mavs', ' Hawks', ' Spurs', ' Nets'), Suppose we have the following two data frames in R: #define first data frameĭf1 <- data. Example: The Difference Between merge() and join() The following example illustrates difference #2 in practice. The join() functions from dplyr preserve the original order of rows in the data frames while the merge() function automatically sorts the rows alphabetically based on the column you used to perform the join. The join() functions from dplyr tend to be much faster than merge() on extremely large data frames.Ģ. There are two main differences between these two functions:ġ. The merge() function in base R and the various join() functions from the dplyr package can both be used to join two data frames together.
